The Answer to AI's 600 Billion Dollar Question
Why AI companies are building out massive datacenters
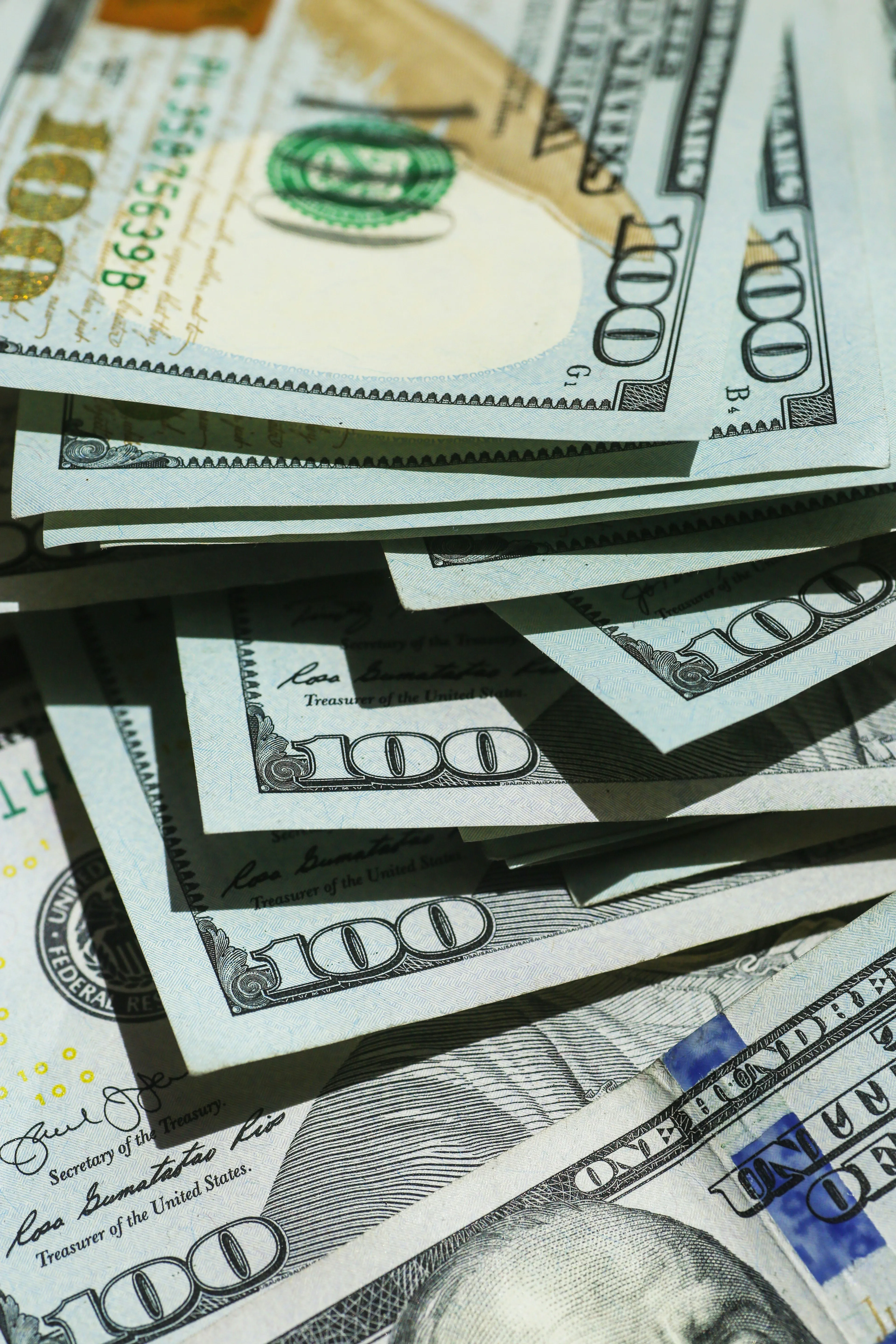
The talks about AI have intensified greatly in the last few years since ChatGPT was first launched and since then generative AI tools like Midjourney, Udio, and Runway have taken the world by storm. Now big companies are pouring countless billions into the rapid buildout of GPU infrastructure.
These new data centers being built, are well suited to running and training ever-larger models. This investment is due to the strong scaling laws at play with machine learning algorithms. Building bigger neural networks and giving them more training time and data to learn from, makes them much better.
The bigger the models get, the more capabilities beyond next token prediction emerge. But all this investment into new compute infrastructure opens up a key question: what is the business use case in the end? Where do companies hope to recuperate the money that they spend now? How is all this GPU power going to deliver value to the customer? Sequoia Capital calls this AI's 600 billion dollar questionβthe question is essentially: what is the use case of AI? And the current use cases are not enough: Generating videos, voices, or "better" search queries, is simply not enough to generate the amount of returns necessary to make the investment worth it. Especially since ad-driven businesses like Google and Meta don't have a good way of injecting ads into the output of machine learning models yet.
To me, there is only a single answer left, through which all of this crazy investment and capital expenditure makes sense. And it's the one all the CEOs are carefully avoiding to mention in their interviews. When asked: "Is this going to replace jobs?", they quickly change the topic, carefully dancing around the question and avoiding answering. But to me, this is the only real reason that would justify their massive investments.
The only world where this sort of scale would make sense is the one where AI is powerful enough that it can outright replace most jobs and where companies are planning to profit from this development (this sort of thinking stems directly from what Leopold Aschenbrenner calls "Situational Awareness").
You certainly would need a lot of compute to automate most human work, and companies are building exactly this. So, my conjecture is simple: All the big companies build out massive GPU inference fleets and new gargantuan data centers because they secretly believe that in the not-too-far future, AI models will be good enough to replace a large chunk of human work.
It's all a massive gamble that hinges on the continuation of the same scaling laws that made LLMs so powerful in the first place. If it's going to pay off, it's going to do so handsomely.
The market for automated knowledge work is essentially unlimited. Right now the only constraint for having more intelligence to bear on a job is the high price of skilled humans. This price comes from the long times that are necessary to "produce" new people capable of doing knowledge work. It takes time for us to learn new skills. It takes even more time before people become exceptionally good at them. This creates bottlenecks that drastically increase the prices of much-in-demand work to a point where bringing more intelligence to bear on a problem is often not economical.
One of the best examples here is probably programming. I've written about this before but I am afraid of AI "coming for my job" and the argument outlined here makes me even more convinced that this will happen.
Sam Altman is trying to raise 7 trillion for consolidating and owning GPU manufacturing capabilities. This only makes sense if he expects AGI to happen. Because in that future, there is an insatiable need for compute: the more computational power you can get your hands on the better.
Because the more compute you can have, the more use cases your AIs can unlock and the more companies you can sell your AI automation to. Or, in an even more sinister turn, the more work you can do yourself. Instead of selling intelligence from AI, just use it to improve your product offering and expand into new markets. Imagine a big company like Microsoft, not just ditching their employees, but starting to compete in all sorts of other business ventures as well. Essentially using an incredibly cheap, almost infinitely scalable team of AI experts to plan and execute their strategies. They would soon start to crush any competitor that doesn't have a similarly sized GPU cluster. It starts sounding like the story of the Omega Team.
So that's it. The answer to the $600B question is simple: automated knowledge work. Now if that actually happens, i.e. if the scaling laws will hold and we will see this sort of automation, is an entirely different question. But the companies and people building out massive data centers certainly believe so.
And I think I'm betting on their side because they are also the ones trying to make sure that this future is coming to pass. They have a massive financial incentive as well as the talent and teams in place to stack the odds in their favor. Making their own future, so that the bet they have made will pay off.